Always an antidote to the hype and bluster around AI and data analytics is time spent at Predict, the annual conference that took place in Dublin earlier this month. According to founder and Creme Global CEO Cronan McNamara, it offers a barometer of what’s really happening in the sector.
“One of the big changes apparent at this year’s event is how technology we have been talking about for years is moving out of the laboratory,” he said, “how it’s now talked of with an eye on real world applications.” True to his word, speakers explored here-and-now use cases in the home, in healthcare, in the food supply chain and in sustainability.
Even speakers in the ‘thinkers’ part of the programme were in pursuit of innovation that had commercial application. Ben Duffy of MTank looked at robotics evolution from the basic challenge of picking up cups to AI with human-like consciousness. He argued that companies had gone bust because they missed the mark on developing AI that was “affordable, useful and robust”, which was leading him to develop multitasking robots with “artificial generalised intelligence”.
Imperfect robots
Trinity College’s Conor McGinn is doing something similar with Stevie, a prototype robot that’s already interacting with old people in US care homes. Addressing the growing crisis of a shortage of carers to look after an ageing population, Stevie multitasks and coexists alongside residents, offering company as well as medical support, acting as an intermediate to facilitate video calls or even run bingo sessions.
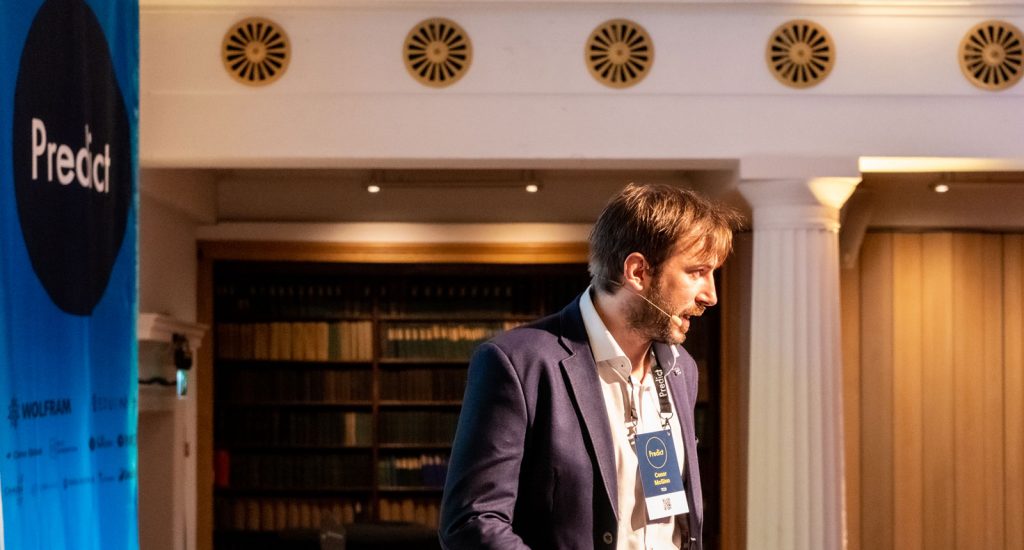
The pilot project is challenging preconceived ideas about robots in the real world, not least that people would be intolerant if they get things wrong. When Stevie’s sound card failed during bingo and he couldn’t articulate the numbers, his audience was very supportive and were giving him encouragement, something that has happened many times since.
Concerns among the research team about failure intolerance turned out to misplaced. “It was only when things went wrong that we got real insights into its [the robot’s] capability of being accepted,” said McGinn. “The fact that it wasn’t perfect, humanised it in a very strange way.” Robots as benign assistants that people care about is a long way from the dystopian vision of AI in science fiction.
Alessandra Pascale, research manager at IBM, sees AI and machine learning as fundamental to making healthcare more efficient and a way to improve patient outcomes. “We have a need for a person-centred approach to care versus the disease-centred approach we see now,” she said. A human behaviour modelling project analyses research to inform patient interventions to help tackle chronic diseases. Another one runs care analytics in the cloud and helps patients self-manage their conditions.
Human and AI interaction
An afternoon session on customer service highlighted one of the more mature market segments, where machine learning in the form of bots is already engaging with people on a regular basis. Ding’s Eric Mehes explained that it’s taking off in contact centres because the cost of acquiring new customers is five times what it takes to retain them. If algorithms can identify and reduce the risk of churn, it’s money well spent.
Not for the first time at Predict, a speaker put pay to “robots are taking our jobs” scare stories. Shane Lynne of Edge Tier said that people are still the best part of customer service and the trick was to combine the nuances of human communication with the speed and accuracy of AI. The need for people was a recurring theme, something The Artomatix’s Eric Risser described as “the glue” in a growing ecosystem of AI services.
Meanwhile on a second stage, scientists from CeADAR, Ireland’s national centre for applied data analytics and AI, were exploring ways to solve societal challenges around sustainability, climate change and city planning. Robert Ross, a senior lecturer at DIT, talked about data scientists using AI for forces of good. There were diverse examples of analytics at work, from predictive maintenance in windfarms to multispectral imaging to improve the quality of grass for farming.
The good news for delegates at Predict is that the organisers want discussion rather than evangelism. Many speakers reminded the audience that analytics projects are complicated, that mistakes are being made that need to be addressed.
Make bad research good
John Elder from Elder Research, a leading US data mining consulting team, brought three decades of statistical analysis experience to the stage. He believes his job, and the role of the growing ranks of data scientists, is to do things better, avoiding the pitfalls of what he describes as a crisis in scientific research.
Results of eminent research in prestigious medical publications like The Lancet cannot be reproduced, prompting the notorious declaration of Dr John Ioannidis that 90 per cent of medical journals are worthless. “Science depends on replication and a finding being real,” said Elder. “We’ve got to get better at solving the technical problems of statistical tests.”
One way to do this, according to Elder, is to use multiple models, to test and test again the veracity of data. Digging down into techniques and algorithms that are fundamental to data science, he made the case for using disparate and competing analytics models to achieve “ensemble averaging” that mitigate the risk of false outputs.
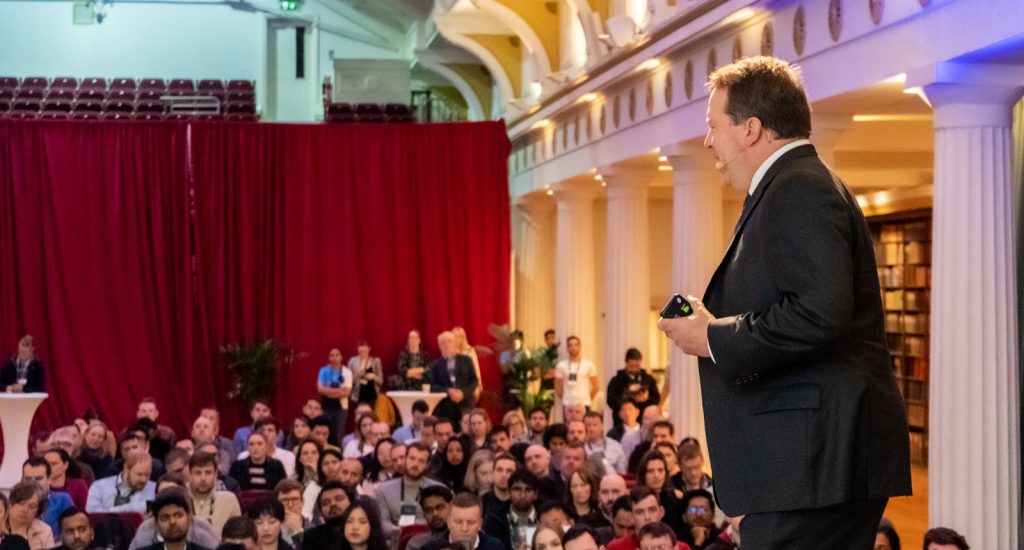
People and bias problems
Perhaps his most salutary warning was that even if you get the algorithms right, even if data analysis reveals a solution to a problem, there is no guarantee that it is will be used. “The great obstacle isn’t as much the technology part of it,” he said. “Where they fail is people actually using them, people actually implementing the beautiful thing you have created.”
There is another challenge the industry must face. Many of the conference delegates would be familiar with the “garbage in, garbage out” mantra of data analytics, that the quality of outputs will always depend on the quality of inputs. It is still fundamental, but made more complicated by a new challenge, “bias in, bias out”.
Susan Leavy, from the Insight Centre for Data Analytics at UCD, highlighted the danger of bias in data, gender bias specifically, and how future focused technology is delivering outputs that threaten to undo hard-earned legislation from the past. She drew attention to stereotypes in AI digital assistants – Alexa and Siri, humble female voices helping out at home, compared to IBM’s male-voiced Watson providing expertise in the workplace – but the problem runs much deeper.
When algorithms are trained on language-based training data, absorbing text that may be historical and inherently biased, misrepresentation will inevitably be perpetuated. She stressed the importance of coming up with models that will root out bias, otherwise we will find ourselves using language about gender that is no better than 19th century fiction.
“We are in danger of going back decades on the advances we have made in human rights and equality,” she warned. Leavy’s presentation was a wake-up call, reminding a room full of data scientists how granular attention to models and algorithms was needed if analytics is to play a part, as many believe it can, in positive societal change.
Conference review written by:
Ian Campbell